Ecology and related fields face a growing divide between empiricists and statisticians. Advances in technology allow empiricists to obtain far more data – be it fine-grain spatial and temporal weather patterns from satellites, drone mapping of plant locations, video recordings of animal behavior, or rapid, inexpensive sequencing of microbial communities. In addition to an increasing stream of data, increasing computational power on top of ongoing research in statistics (particularly machine learning) has dramatically increased the number of potential statistical tools researchers can use to understand their data. Yet ecologists have as much – in truth, far more – domain-specific reading and learning to do than they did when t-tests were state of the art. As a consequence, there is often a disconnect between ecological studies and the most appropriate statistical tools. I am passionate about using statistical and computation tools to bridge the gap between data and questions.
My research program investigates a range of questions in ecology and evolutionary biology by bringing statistical and computational tools to collaborations with experimental biologists. This includes adapting and developing statistical methods (e.g. Teller et al. 2016, Edwards and Crone in revision), linking data and theory through simulation and mathematical models (Edwards et al. 2018, Edwards and Yang in press), and providing the statistical and computational support to implement existing methods (Bonoan et al in review, ongoing collaborations in bird physiology, soil science, and linguistics). Some of these projects are shaped or inspired by collaborations with empiricists who need a data scientist to link their data to questions and patterns (e.g. my work on demography, phenology, and movement of at-risk butterflies).
In general, I spearhead projects addressing three urgent questions in community ecology: (a) how do plants defend themselves from herbivores, and how do the strategies they employ structure plant and insect communities?, (b) how do fine-grain weather patterns and events influence population dynamics and phenology?, and (c) what structures microbiome communities, and how can we harness existing data to better manage human microbiomes and indoor ecology? While these questions cover disparate systems, they are linked by a similar statistical problem: in each case, we generally have high dimensional (“wide”) predictor data, but either limited or potentially problematic measures of responses. How can we best infer the underlying drivers of the patterns that we see?
At-Risk Butterflies
While there is an ongoing conversation about the extent of insect declines, numerous studies have documented that many insect species are declining. at the same time, many species are shifting their phenology – the timing of their life history events – in response to a changing climate.
Working with Prof. Elizabeth Crone, I have developed a novel use for Generalized Linear Mixed Models (GLMMs) (Edwards and Crone, in revision), which allows us to estimate timing and population abundance from sparse count data of at-risk insects. In a collaboration between Professors Elizabeth Crone, Cheryl Schultz, and Rachael Bonoan, we used this method to provide the first evidence that the at-risk Fender’s Blue butterfly has been responding well to conservation management. In collaboration with Elizabeth Crone and Cheryl Schultz, I am now implementing these methods to estimate population and phenology changes of more than 30 species of at-risk butterflies using long-term scientist and community scientist observations.
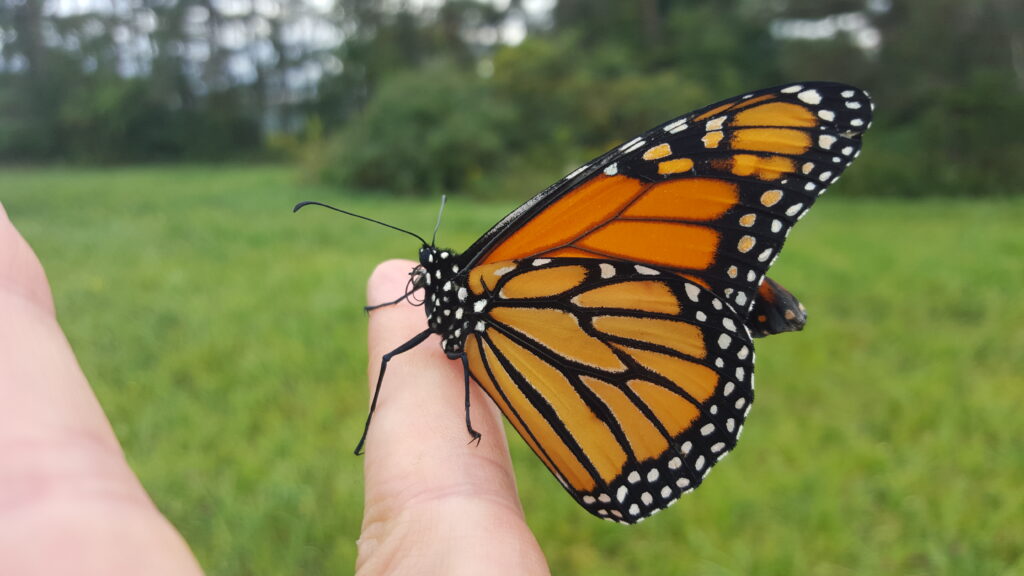
I have also adapted our statistical methods method to uncover the dramatic shift in yearly range expansion of the western Monarch Butterfly, using thousands of citizen-science observations. In the 1990s, there were millions of Western monarch butterflies, yet in the last few years there have been only ~30,000 individuals. This decline has involved both a generally negative population growth for the last thirty years, and several distinct crashes included an 85% decline in the winter of 2017/2018. Working with Elizabeth Crone, Cheryl Schultz, and Emma Pelton, I have found that yearly expansion rates (starting from the overwintering sites on the coast of California, expanding out through the Western US and Canada) were hugely reduced after the 2017/2018 crash, but not previous crashes. Among other things, this was seen in the almost complete absence of monarch butterflies in the state of Washington in 2018 and 2019, even though they were fairly prevalent in the summers of previous years. Here is a 15 minute talk I gave on this work.
Plant Defense Strategies
Like unfortunate characters in a grotesque horror film, plants are rooted in the ground as herbivores eat them alive. Rather than fleeing, as a gazelle might dash from a cheetah, plants instead must rely on defense strategies survive. Plant defense strategies are complicated, and plants often employ multiple defensive traits (e.g. toxins, hairs, latex, silica, etc) simultanously. While a number of hypotheses have been proposed to explain why plants would use the combinations of defenses that they do, testing these hypotheses has been difficult. Ultimately, I would like to explain how plant defense strategies structure plant and insect communities, but first we must understand how plant defense strategies work. To do so, I have been collaborating with Professors Stephen P. Ellner and Anurag Agrawal.
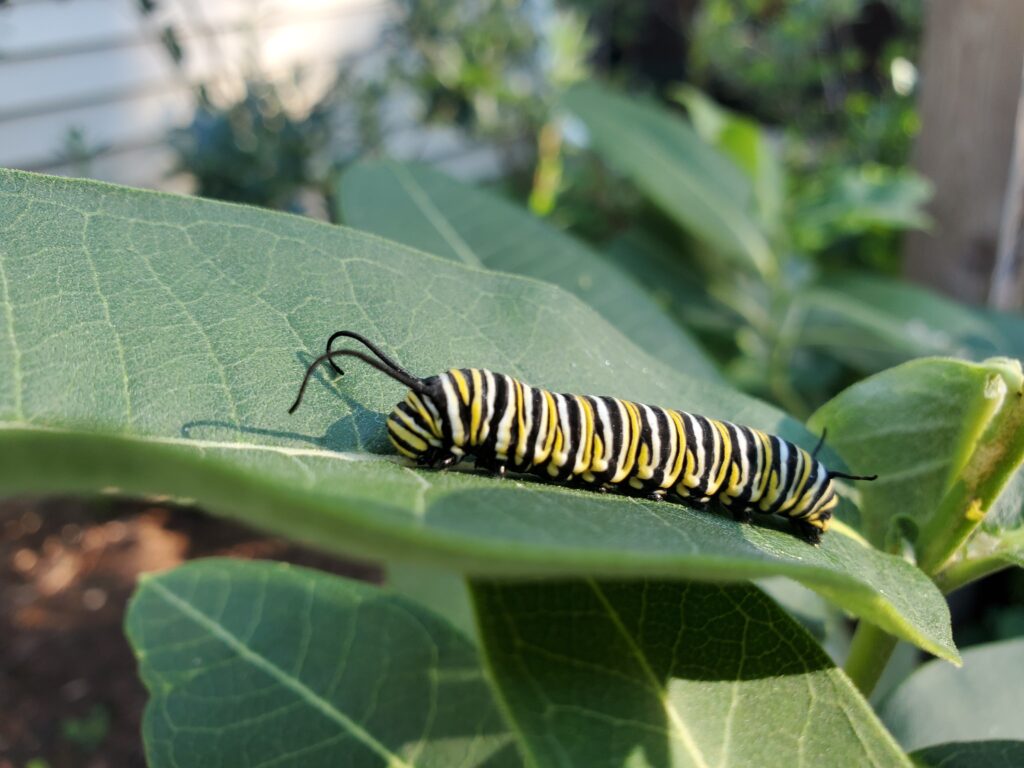
Employing a combination of field experiments and statistical modeling, I set out to test two of the most popular hypotheses: that defense traits can interact synergistically (so plants might employ traits that boost each others’ efficacy), and/or that the value of traits are context-dependent (so plants might bet-hedge by employing several). I carried out bioassays of monarch butterfly (Danaus plexippus) and swamp milkweed beetle (Labidomera clivicollis) larvae on common milkweed (Asclepias syriaca). This work is nearing submission, but the short version is that the patterns we detected largely favored the idea of context-dependent defense traits, and we did not detect the previously hypothesized synergies.
To look for more general patterns, I developed a dynamical model of plant and herbivore growth, with plant defenses limiting herbivore performance and thus indirectly improving plant performance. By parameterizing this with a variety of empirical studies, I can explore a wide range of possible plant and insect pairings. This work is still in progress, but it appears that rather than one mechanism or another explaining why plants benefit from combining defense traits, these mechanisms themselves act synergistically – a little bet-hedging and a little trait synergy can easily explain the prevalence of mixed defense strategies, even though bet-hedging alone or trait synergies alone would have to be extreme to favor mixed defense strategies.
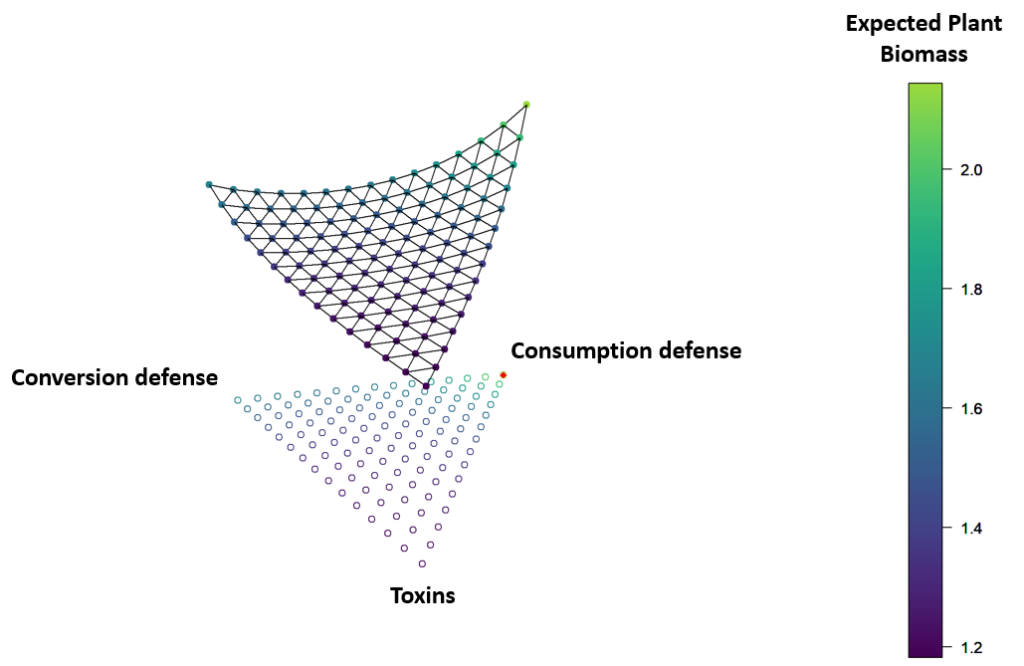
Building a theoretical framework for microbiome data
With the development of modern genomic and metagenomic tools, microbial ecologists are gathering a wealth of data of microbial communities. However, analyses of these data remain descriptive, limited in part by a lack of appropriate statistical tools to link genomic data with the rich understanding of community assembly and coexistence developed mostly from larger organisms (particularly plants). Starting in December, I will lead analyses of existing data collected by the lab of Professor Benjamin Wolfe (also collaborating with Elizabeth Crone). My goal is to explore the intersections of this rapidly growing microbiome data and the rich body of ecological coexistence literature. The fact that I get to do so while exploring cheese (and kimchi, kombucha, sauerkraut, and other fermented foods) is just a side benefit.
